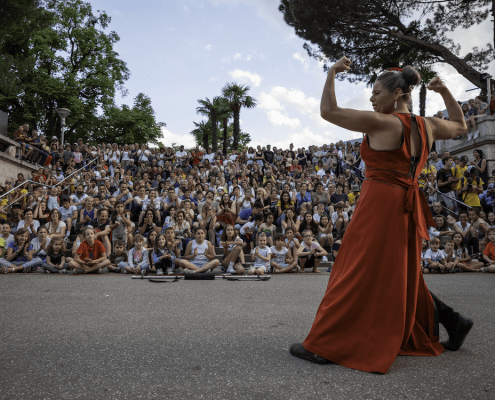
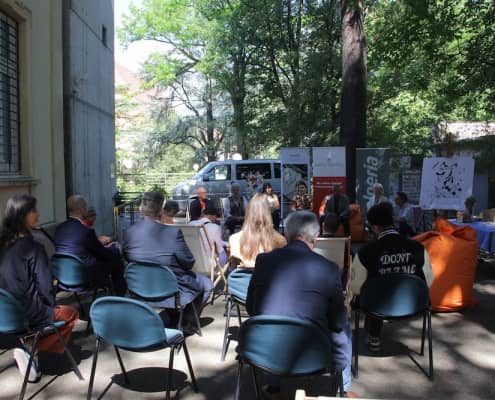
Wir und ausgewählte Dritte setzen für technische Zwecke und, mit Ihrer Einwilligung, für andere Zwecke Cookies und ähnliche Technologien ein, so wie in der Cookie-Richtlinie beschrieben. Ablehnung kann entsprechende Funktionen unverfügbar machen. Ihre Einwilligung können Sie jederzeit erteilen, verweigern oder widerrufen. Verwenden Sie den „Zustimmen“-Button um der Verwendung solcher Technologien zuzustimmen. Verwenden Sie den „Ablehnen“-Button oder schließen Sie diesen Hinweis um fortzufahren, ohne zuzustimmen.
ZustimmenAblehnenEinstellungen
Unbedingt notwendige Cookies sollten jederzeit aktiviert sein, damit wir deine Einstellungen für die Cookie-Einstellungen speichern können.
Sie haben die Möglichkeit zu verhindern, dass von Ihnen hier getätigte Aktionen analysiert und verknüpft werden. Dies wird Ihre Privatsphäre schützen, aber wird auch den Besitzer daran hindern, aus Ihren Aktionen zu lernen und die Bedienbarkeit für Sie und andere Benutzer zu verbessern.
Facebook - Twitter - Google+ - Youtube - Vimeo
Beim Besuch einer Webseite werden Cookies sowohl von der besuchten Seite ("Eigentümer"), als auch von anderen Organisationen ("Dritten") gesetzt. Besonders hervorzuheben sind die "Social Plugins" wie Facebook, Twitter, Google+, Youtube und Vimeo. Es handelt sich dabei um Bereiche der besuchten Webseite, die von den Seiten Dritter generiert und in die besuchte Webseite integriert werden. Die häufigste Verwendung von Social Plugins zielt auf den Austausch von Inhalten mit sozialen Netzwerken ab. Wollen Sie vermeiden, daß Facebook – Twitter – Google+ – Youtube – Vimeo über diese Webseite Daten über Sie sammelt, müssen Sie sich vor Ihrem Besuch unseres Internetauftritts bei Facebook – Twitter – Google+ – Youtube – Vimeo ausloggen.
Diese Plugins beziehen sich auf die Übertragung der Cookies von und zu Webseiten Dritter. Diese Informationen von "Dritten" werden in den entsprechenden Regelungen verwaltet, die bitte zu beachten sind.
Mehr Informationen über unsere Datenschutzbestimmungen.